Research Snapshot: Skills-First Transformation: Building a Skills Taxonomy Leveraging AI
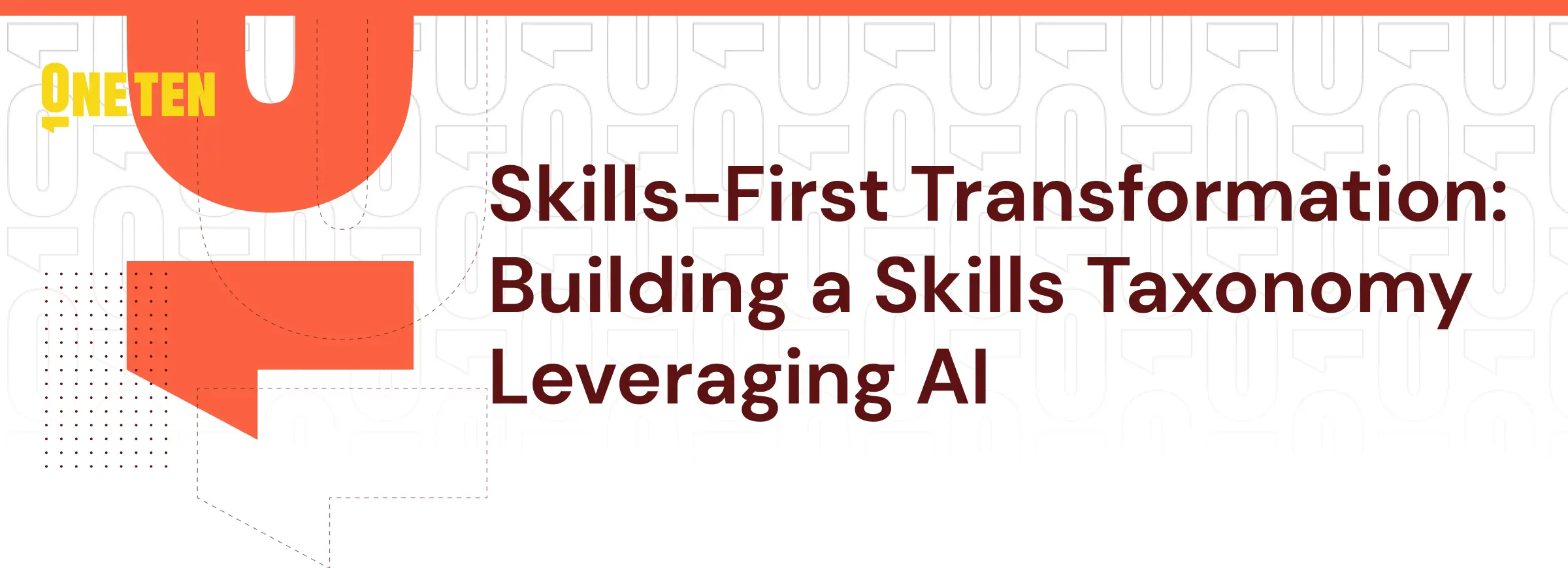
03.28.2025
What is a Skills Taxonomy and Why Is It Essential for Building a Skills-First Workforce?
A skills taxonomy is a framework that organizes and groups skills into a hierarchy, helping organizations define, track, and understand the skillsets of their workforce, identify skill gaps and facilitate workforce development. This framework provides a shared language for classifying both technical and durable (soft) skills based on factors such as proficiency levels, job function relevance, and market trends. Developing a skills taxonomy is central to a skills-based strategy, serving as a foundational data-driven resource for workforce management, internal mobility, and succession planning.
Many organizations find it challenging to understand what a skills taxonomy is and how to build one effectively. This research snapshot clarifies the concept and essential components of a skills taxonomy, showing how it enables organizations to effectively track and manage the skillsets of their workforce to enhance agility and drive organizational success.
Leveraging Skills Taxonomies to Drive a Skills-First Workforce
The modern workplace is undergoing rapid change with nearly 45% of current workplace skills expected to evolve or transform entirely within the next five years. To address this challenge, organizations are turning to skills taxonomies to anticipate shifts and proactively identify skill gaps. These taxonomies play a crucial role in supporting HR functions across the talent lifecycle, which are becoming more skills-focused in response to these workforce changes.
Identifying required skills is essential for workforce transformation, yet 53% of HR leaders report that the primary barrier to achieving a digital-ready workforce is precisely this challenge. Currently, only 45% of companies have even a basic skills library-a preliminary step toward a fully developed skills taxonomy-with just 14% formally monitoring market demands and only 51% doing so informally.
The complexity and manual effort involved in building and maintaining a comprehensive skills taxonomy can overwhelm HR teams, with limited HR capacity cited as the top obstacle to becoming a skills-powered organization. This gap underscores the need for organizations to leverage skills taxonomies to help them navigate these challenges more efficiently and keep their workforce competitive and adaptable in a rapidly evolving landscape.
The advent of generative Al introduces a tech-enabled, hybrid approach to building a comprehensive skills taxonomy that reduces the complexity and resource demands involved in its development. By automating key aspects, Al makes it feasible for organizations to efficiently identify, monitor and update required skills, empowering HR teams to build a skills-powered workforce.
The Role of AI in Skills Taxonomy Development
Recent developments in generative AI (GenAI) have enabled the creation of tools like SkillsGPT and WorkforceGPT, which streamline the process of analyzing diverse data sources such as skill frameworks (e.g., O*Net) and labor market data to keep taxonomies updated frequently and efficiently. Organizations can leverage these AI tools to quickly identify skill gaps, recommend tailored upskilling pathways, and forecast emerging skills by drawing insights from both internal workforce data and external labor market trends.
While AI can automate tasks such as generating action verbs to describe skills or organizing skills ontologies, human expertise remains essential to ensure accuracy. Human expertise is critical for complex processes such as verifying data accuracy and refining the taxonomy framework based on inconsistencies that can be generated by AI. This collaboration between AI and human subject matter expertise is vital to achieving an accurate and effective skills taxonomy. Key use cases demonstrating this collaboration include:
AI Use Case | AI Capabilities | Human Intervention Required |
---|---|---|
Automated Skills Taxonomy Creation | Automates the creation of skills taxonomies by analyzing organizational data, occupations, and labor market trends to build adaptive, data-driven taxonomies. | Review and validate the taxonomy to ensure accuracy and relevance for the organization. |
Identification, Standardization and Mapping of Skills | Scans roles to identify and recommend essential skills, and standardize skills taxonomies for a unified understanding across the HR ecosystem | Ensure skills mapping aligns with specific organizational needs and adapt as necessary. |
Skill Gap Analysis | Continuously assesses workforce skills to identify gaps, informing targeted reskilling efforts. | Review and verify identified gaps to ensure alignment with organizational goals. |
Personalized Reskilling Pathways | Develops individualized learning paths by analyzing skills gaps, mapping gaps and recommending reskilling pathways. | Verify and adjust reskilling pathways to meet organizational standards and objectives. |
Continuous Adaptation of Learning/ Development Programs | Dynamically updates training programs to align learning content with evolving skills taxonomies, ensuring workforce relevance. | Ensure updated training content is relevant and applicable. |
Predictive Workforce Planning | Predicts future skills requirements, helping organizations prepare for market driven changes. | Validate forecasts and modify workforce planning strategies as needed. |
AI-Driven Skills Development at Johnson & Johnson: A Case Study
In 2020, Johnson & Johnson (J&J) was on a mission to transform its workforce into a digital-first organization, aiming to equip employees with the skills needed for innovation and process modernization. The company faced a primary challenge: assessing the existing skills of its workforce, identifying gaps and enabling skill development using an approach that could capture future-ready capabilities. Traditional skill assessment methods were outdated and incomplete, prompting J&J to implement a new, Al-driven strategy. Key steps included:
- Proof of Concept (PoC) Development: Partnering with IBM, J&J launched a PoC using machine learning (ML) to infer a set of core skills within a pilot group of employees.
- Building the MySkills Platform: Leveraging insights from the Poe, J&J developed the MySkills platform, powered by Al, to assess and enhance technical and leadership skills. This platform integrates data from HR systems, learning management systems and project management tools, using ML to infer skill levels across its global workforce.
- Defining Future-Ready Skills and Data Sources:J&J's team, in collaboration with IBM, identified 41 essential future ready skills. They then selected robust data sources to train the Al model for accurate skill inference.
- Addressing Ethical AI Use: To ensure ethical Al practices, the MySkills team established guidelines and hosted Al ethics workshops. Transparency was prioritized, allowing employees to control their data, opt-out, and even challenge inferred skill levels.
- Expanding Beyond Technical Skills: Expanding beyond technical competencies, J&J added leadership skill assessments by integrating data from its peer recognition platform, enabling the Al to infer durable (soft) and leadership skills.
- Effective Change Communication: To foster employee buy-in, J&J held town halls and roundtables, clearly communicating that MySkills was designed for career development, not performance evaluation.
Through the MySkills platform, J&J successfully built a dynamic and scalable skills taxonomy that supports strategic workforce planning and talent development initiatives. By combining Al capabilities with strong data governance, ethical considerations and continuous human oversight, J&J harnessed the potential of Al to create a future-ready workforce, offering a model for other organizations aiming to implement similar initiatives.
The Path to a Skills-First Workforce Leveraging a Robust Skills Taxonomy
A robust skills taxonomy is a building block for organizations seeking to effectively build a skills-first workforce. Al tools show great promise for efficiently scaling skills-first planning and development by automating skill identification, monitoring market demand and managing skills libraries. These tools allow organizations to overcome the barriers of manual management of skills taxonomies, such as the complexity of identifying emerging skills and responding to rapidly changing labor market trends.
Ultimately, combining Al with human expertise will be essential in building a skills taxonomy that prepares organizations for a future-ready, skills-driven workforce. To help you put these ideas into practice, click here to access: OneTen’s Evidence-Based Guide for Building a Skills Taxonomy Using AI.Â
Download the PDF